Thoughts move through our minds like a train – each one connected to the next to form a continuous chain. At times this train speeds efficiently toward a destination, while other times it meanders aimlessly without a clear track. Chain of thought prompting works like a conductor that helps guide this train of ideas by posing thoughtful questions to keep the cars linked and headed in a productive direction. It’s a way of building an agile chain of reasoning by steering our own thought processes. Just as connecting railcars allows the train to cover more conceptual ground, linking each idea to the next can transport our thinking farther than if thoughts merely spurred at random. With practice as the conductor, we can use chain of thought prompting to actively explore topics more deeply and reach new insights. All aboard for this journey to improve reflective reasoning.
The Purpose Behind the Prompts
The goals of using a chain of thought include organizing one’s thinking process, identifying logical connections between ideas, and reaching a well-reasoned conclusion. The benefits of employing a chain of thought include improved problem-solving skills, enhanced critical thinking abilities, and the ability to communicate ideas more effectively.
Constructing the Chains of Questions
Creating effective prompting chains that link ideas can be a valuable skill for various tasks, including brainstorming, problem-solving, and writing. Here are some tips for coming up with effective prompting chains:
- Start with a Clear Objective: Clearly define the objective or the main idea you want to explore. This will provide a focus for your prompting chain and help guide the direction of your thoughts.
- Use Open-Ended Questions: Begin with open-ended questions that encourage exploration and elaboration. These questions should prompt thinking about different aspects of the main idea and lead to related sub-ideas.
- Encourage Divergent Thinking: Prompting chains should encourage divergent thinking, allowing for the generation of multiple ideas and perspectives. Avoid closed-ended questions that limit the scope of exploration.
- Link Ideas with Associations: As you progress through the prompting chain, link ideas by finding associations between them. This can be done by identifying similarities, differences, or causal relationships between the ideas.
- Explore Different Perspectives: Prompting chains can be more effective when they consider various perspectives. Encourage thinking from different angles, such as emotional, logical, practical, or creative viewpoints.
- Use Visual Aids: Consider using visual aids such as mind maps or diagrams to visually represent the prompting chain. This can help in organizing and connecting ideas more effectively.
- Iterate and Refine: After generating a series of prompts and linked ideas, iterate through the chain to refine and expand upon the connections. This iterative process can lead to deeper insights and more comprehensive chains.
By following these tips, individuals can develop effective prompting chains that facilitate the exploration and linkage of ideas, leading to richer and more nuanced understanding of the main concept.
Examples in Practice
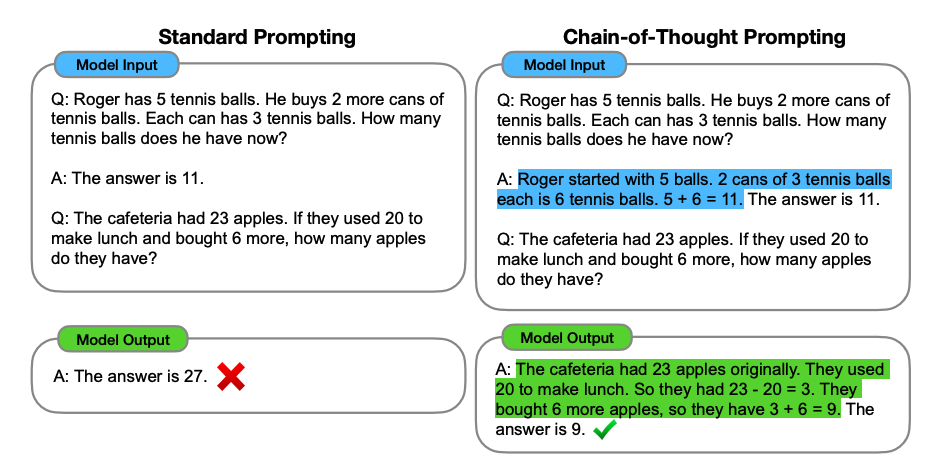
Prompt 1: What is your favorite subject in school?
Possible Response: I really enjoy math class.
Prompt 2: What about math do you enjoy the most?
Possible Response: I like that there are clear steps to solve problems and get the right answers.
Prompt 3: How do you feel when you get stuck on a hard math problem?
Possible Response: I feel frustrated at first, but I know if I keep trying different strategies I’ll figure it out.
Prompt 4: What strategies do you use when you get stuck?
Possible Response: I go back and double check my work, look at examples from the book, or ask my teacher for a hint.
Prompt 5: When have you used math strategies in your life outside of school?
Possible Response: Well one time I was baking cookies and had to double the recipe – I used fractions to figure out the new measurements.
In this chain, the first question sparks an interest area, then each follow up question builds off the previous response to guide reflective thinking. It explores reasons behind liking math, reactions to challenges, and how math applies more broadly. The prompts aim to keep a continuous flow while uncovering new angles on the initial topic. This helps the speaker think more multidimensionally through chained reasoning.
When Chains Break Down
When the line of thinking gets disrupted, it’s important to take a step back and reassess the situation. Here are some steps to consider:
- Pause and Reflect: Take a moment to pause and reflect on the disruption. It’s essential to acknowledge that disruptions are a natural part of the thinking process.
- Identify the Disruption: Try to pinpoint the exact cause of the disruption. It could be due to external factors, internal distractions, or a lack of clarity on the topic.
- Revisit the Basics: Sometimes, going back to the basics of the topic or problem can help in re-establishing the train of thought. This can provide a fresh perspective and help in overcoming the disruption.
- Seek Input from Others: Discussing the problem with a colleague or mentor can often provide new insights and help in overcoming the disruption.
- Break Down the Problem: If the disruption is due to a complex problem, breaking it down into smaller, more manageable parts can make it easier to tackle.
- Utilize Tools and Techniques: Depending on the nature of the disruption, various tools and techniques such as mind mapping, brainstorming, or visualization can be employed to regain focus.
- Take a Break: If the disruption persists, taking a short break can be beneficial. Stepping away from the problem for a while and returning with a fresh mind can often lead to new perspectives.
Remember, disruptions are a normal part of the thinking process, and overcoming them often leads to deeper understanding and insight.
Based on the provided information, the concept of “chain-of-thought prompting” is discussed, which is a method for enhancing reasoning in language models. It involves augmenting each exemplar in few-shot prompting with a chain of thought for an associated answer. The study shows that chain-of-thought prompting is an emergent ability of model scale and enables large language models to solve challenging math problems. It also compares favorably to prior state of the art on various datasets. The study also includes an ablation study with variations of chain-of-thought prompting, such as “equation only” and “variable compute only” prompting.
Proof Chain of Thought Works
This paper provides evidence that chain-of-thought prompting works to elicit reasoning in large language models. The paper “Chain-of-Thought Prompting Elicits Reasoning in Large Language Models” explores how generating a chain of thought significantly improves the ability of large language models to perform complex reasoning. The study shows that such reasoning abilities emerge naturally in sufficiently large language models via a simple method called chain-of-thought prompting, where a few chain of thought demonstrations are provided as exemplars in prompting. The experiments on three large language models show that chain-of-thought prompting improves performance on a range of arithmetic, commonsense, and symbolic reasoning tasks. The empirical gains can be striking, with chain-of-thought prompting achieving state-of-the-art accuracy on challenging benchmarks such as the GSM8K benchmark of math word problems.
The study provides empirical evidence that chain-of-thought prompting outperforms standard prompting, sometimes to a striking degree. For instance, on the GSM8K benchmark of math word problems, chain-of-thought prompting with PaLM 540B outperforms standard prompting by a large margin and achieves new state-of-the-art performance. The study also includes an ablation study that explores different variations of prompting, confirming the effectiveness of chain-of-thought prompting in facilitating reasoning in language models.

The evidence from the study supports the effectiveness of chain-of-thought prompting in eliciting reasoning in large language models, particularly for tasks such as arithmetic reasoning, commonsense reasoning, and symbolic manipulation.
Therefore, based on the evidence from the study, there is proof that chain-of-thought prompting works to elicit reasoning in large language models, and the diagrams provided in the paper illustrate how chain-of-thought prompting enables large language models to tackle complex arithmetic, commonsense, and symbolic reasoning tasks.